Development of a Deep Learning Toolkit for MRI-Guided Online Adaptive Radiotherapy
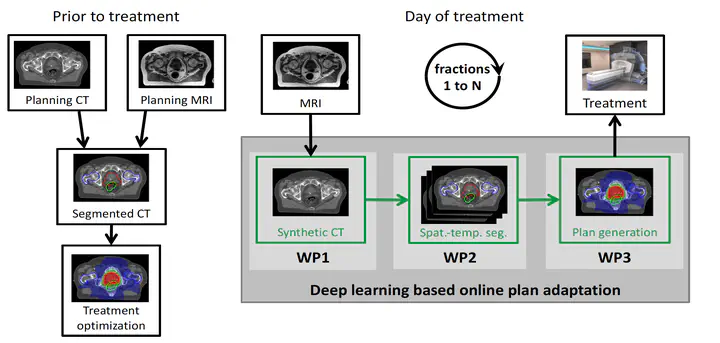
In Germany close to 500,000 new patients are diagnosed with cancer every year. More than half of the patients undergo radiotherapy as part of their treatment. In-room imaging, in combination with modern external beam radiotherapy techniques, enables tight adjustment of the delivered dose to the target. However, the full potential of these techniques is currently not exploited. One of the reasons for this is the presence of anatomical changes. For the vast majority of patients in today’s radiotherapy, anatomical alterations are considered by introducing safety margins around the tumor volume during treatment planning, which increases the irradiated volume, the dose burden to organs at risk (OARs), and eventually limits the applicable dose.
An improved treatment can be realized by online adaptive radiation therapy (ART): instead of applying the same irradiation plan throughout the entire course of treatment, the treatment is optimized at each irradiation session on basis of the daily anatomy in treatment position, as inferred from in-room imaging. Great efforts have been made to realize the integration of magnetic resonance imaging (MRI) as in-room imaging modality, but only during the last few years integrated MR-linacs became clinically available at few academic institutions. The superior soft-tissue contrast allows for accurate visualization of targets and OARs, which has allowed basic online ART workflows to be implemented. The time overhead associated with the generation of a synthetic-CT from MRI and a re-delineation of the patient for treatment plan optimization greatly limits applicability to all patients.
The goal of this project is to tackle the main bottlenecks of online plan adaptation: synthetic CT generation and organ delineation. By combining our respective expertise in radiotherapy and artificial intelligence, we aim at proposing deep-learning-based solutions to build a toolkit to tackle both of these challenges. In the case of synthetic CT generation, we will use generative adversarial networks with hybrid paired-unpaired training paradigms, additionally exploiting novel embedding loss functions to ensure geometric fidelity. Alternatively, disentangled representations will be investigated. For segmentation we aim at exploiting the information from previous fractions by performing spatio-temporal segmentation using longitudinal spatial memory networks. Finally, we aim at evaluating the benefits from these approaches by performing an evaluation of their clinical impact in terms of plan optimization, including physician grading of delineations and robustness assessment. The time benefits, including potential corrections to segmentations and synthetic CT, will also be quantified.